School dates, venue and topics
The school will take place on 27-29 June at the University of Southampton, England, UK. The school is planned to be in-person only, no live streaming will be provided. There will be two courses focusing on different sides of the continuous optimization world, specifically on
1. Distributionally robust optimization
2. Error bounds in optimization
The lectures on robustness will be delivered by Daniel Kuhn, on error bounds by Anthony Man-Cho So. The first two days will included about 6 hours of lectures plus coffee breaks. An excursion to Winchester is planned for Sunday 29.
Attendance
Attendance is free of charge but with a mandatory registration. Lectures are particularly suited for PhD students and young researchers to provide them with the chance of attending two high level courses on continuous optimization, but the school is open to everyone wishing to participate. To register fill this form by 5 May (please, register only when you are sure you are coming: the available classroom is large, so everyone should have a place – in the very unlikely event of too many registrations the priority will not be set upon early booking).
Timetable
Friday 27 June – Daniel Kuhn
Saturday 28 June – Anthony Man-Cho So
9:00 – 10:30 Lecture I
10:30 – 11:00 Coffee break
11:00 – 12:30 Lecture II
12:30 – 14:00 Lunch break
14:00 – 15:30 Lecture III
15:30 – 16:00 Coffee break
16:00 – 17:30 Lecture IV/Discussion
Sunday 29 June – Excursion to Winchester
The courses
Distributionally Robust Optimization
by Daniel Kuhn
Distributionally robust optimization (DRO) studies decision problems under uncertainty where the probability distribution governing the uncertain problem parameters is itself uncertain. A key component of any DRO model is its ambiguity set, that is, a family of probability distributions consistent with any available structural or statistical information. DRO seeks decisions that perform best under the worst distribution in the ambiguity set. This worst case criterion is supported by findings in psychology and neuroscience, which indicate that many decision-makers have a low tolerance for distributional ambiguity. DRO is rooted in statistics, operations research and control theory, and recent research has uncovered its deep connections to regularization techniques and adversarial training in machine learning. This course will present the key findings of the field in a unified and self-contained manner.
Error Bounds in Optimization
by Anthony Man-Cho So
Many contemporary applications in science and engineering give rise to optimization problems that have specific structures, and it is often observed numerically that various iterative methods for solving these structured problems enjoy fast convergence rates. Can we theoretically show that these methods are able to exploit problem structures to achieve fast convergence? To address this question, a powerful approach is to examine the problem structure through the lens of error bounds or the Kurdyka-Lojasiewicz (KL) inequality. Roughly speaking, an error bound provides an estimate of the distance to the solution set of a problem, while the KL inequality provides an estimate of the growth of the objective function of a problem. These estimates yield information on how the iterates generated by different methods progress, thereby allowing one to study the convergence behavior of these methods. In this course, we first introduce the error bound-based and KL inequality-based frameworks for convergence rate analysis of iterative methods. Then, we showcase a number of application scenarios of these frameworks. The scenarios are drawn from applications in computational economics, machine learning, signal processing, and statistics, so as to demonstrate the wide applicability of the said frameworks. Lastly, we discuss the interplay between error bounds and the KL inequality, which offers further insights into how these two tools capture the structure of an optimization problem.
The lecturers
Daniel Kuhn (École Polytechnique Fédérale de Lausanne)
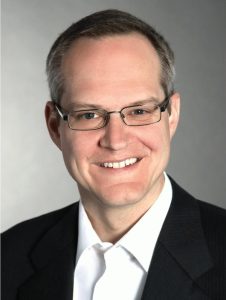
Anthony Man-Cho So (The Chinese University of Hong Kong)
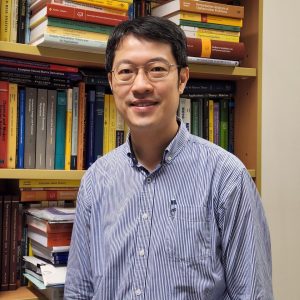
Anthony Man-Cho So is currently Dean of the Graduate School, Deputy Master of Morningside College, and Professor in the Department of Systems Engineering and Engineering Management at The Chinese University of Hong Kong (CUHK). His research focuses on optimization theory and its applications in various areas of science and engineering, including computational geometry, machine learning, signal processing, and statistics.
Dr. So is a Fellow of IEEE and a Fellow of the Hong Kong Institution of Engineers. He is the recipient of a number of research and teaching awards, including the 2024 INFORMS Computing Society Prize, the SIAM Review SIGEST Award in 2024, the 2018 IEEE Signal Processing Society Best Paper Award, the 2015 IEEE Signal Processing Society Signal Processing Magazine Best Paper Award, the 2014 IEEE Communications Society Asia-Pacific Outstanding Paper Award, and the 2010 INFORMS Optimization Society Optimization Prize for Young Researchers, as well as the 2022 UGC Teaching Award (General Faculty Members Category) of the University Grants Committee of Hong Kong. Dr. So currently serves on the editorial boards of Journal of Global Optimization, Mathematical Programming, Mathematics of Operations Research, Open Journal of Mathematical Optimization, and Optimization Methods and Software. He has also served as an associate editor of IEEE Transactions on Signal Processing and SIAM Journal on Optimization, as well as the Lead Guest Editor of IEEE Signal Processing Magazine Special Issue on Non-Convex Optimization for Signal Processing and Machine Learning.
Organisation