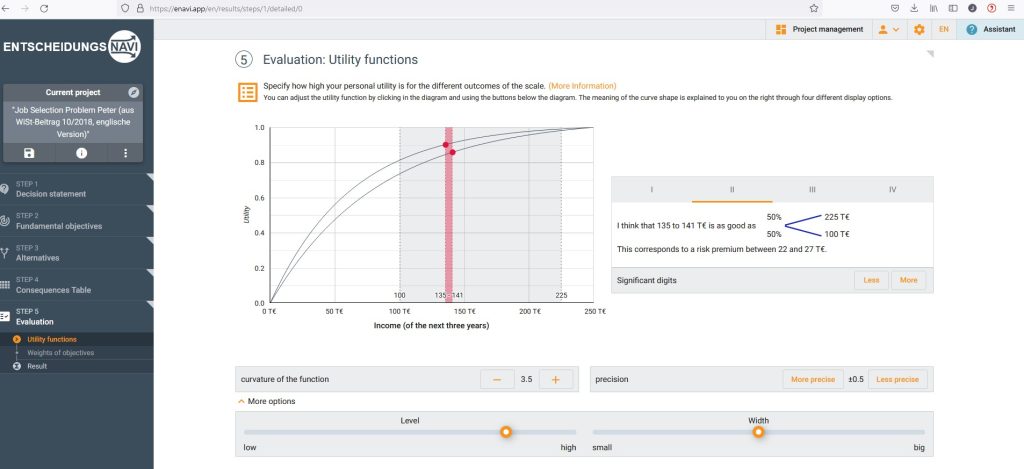
Mendy Tönsfeuerborn (presenting author), RWTH Aachen University, Decision Theory and Financial Services Group, 52062 Aachen, Germany
Johannes Ulrich Siebert (presenting author), Management Center Innsbruck, Department Business and Management, 6020 Innsbruck, Austria
Rüdiger von Nitzsch, RWTH Aachen University, Decision Theory and Financial Services Group, 52062 Aachen, Germany
October 13th, 2022,
Meeting-ID: 824 4319 4473 // Code: 1
https://us02web.zoom.us/j/82443194473?pwd=V0lmWFRRL0R6Tm56QTdRUHNoMWI3QT09
Comment: In case of technical problems, please visit before the start of the meeting: https://www.euro-online.org/websites/bor/behavioral-operation-research-brown-bag-seminar-series/.
Abstract:
Multi-attribute utility theory (MAUT) is broadly used to evaluate alternatives. With the help of partial utility functions, decision makers can express their preferences in decision situations. For a utility function, the most straightforward shape is linear; however, a variety of other, more complicated shapes are suggested in the literature. Researchers have developed more and more sophisticated methods for several decades to elicit preferences precisely. They work on the assumption that the more precisely the preferences are modeled, the better the results of the decision analysis will be. If these time-intensive methods are applied, the utility functions are often non-linear. We found only scarce empirical evidence analyzing to what degree the precise elicitation of preferences is worth the effort.
To fill this gap, we investigate the extent to which linearization of non-linear utility functions leads to rank shifts, especially of the best-ranked alternative. We analyzed 1,959 real-world decisions in the decision support tool Entscheidungsnavi. In the decision frontend, the Entscheidungsnavi provides users with substantial support based on value-focused thinking to help them define and structure their decisions. In the decision backend, MAUT is used to elicit and aggregate preferences. The participants were trained to use the Entscheidungsnavi and spent several hours making their decision. Therefore, it can be assumed that they articulated their preferences accordingly. Most participants (97.40%) used non-linear utility functions and identified between 3 and 9 alternatives. We calculated the rankings for the participants’ stated preferences and the rankings that resulted when all utility functions were linearized. Our analysis reveals that in 85.55% of cases, using linear utility functions instead of non-linear utility functions did not affect the best-ranked alternative. Moreover, we demonstrated that the linearization of the highest weighted objective has a higher impact on the best alternative than linearizing the least weighted. On top of that, we provide regression lines with which the decision maker can assess and evaluate the risk of a rank shift by simplifying the determination of one or more utility functions through linearization.
Why should you join?
We provide unique insights about the implications of using non-linear utility functions.
We provide recommendations about the level of effort, which should be spent to elicit preferences in certain types of decision situations.
We assume that there will an interesting and controversial discussions about using non-linear utility functions.